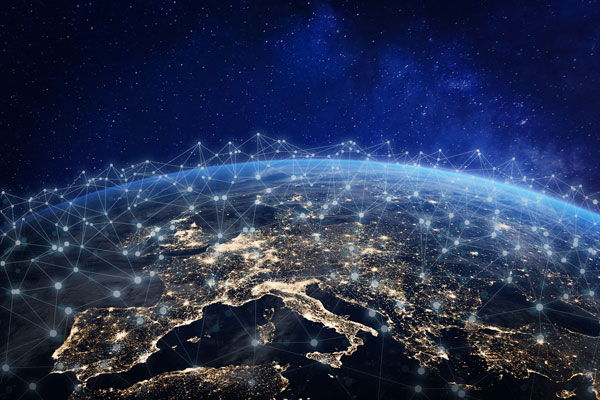
EXPLANT
Leveraging Ensemble Explainable AI for Enhanced Visual Interpretability in Plant Disease Detection
Résumé du projet
This project develops an ensemble approach to explainable AI for plant disease detection, combining techniques like CAM, Grad-CAM, and Saliency Maps. By improving model interpretability, the approach aims to provide clearer, more accurate explanations of AI predictions. This will help farmers and agronomists better understand diagnoses, enhancing trust in AI systems and improving plant health management.
Résultats attendus
The project focuses on developing an ensemble approach to explainable AI techniques – such as CAM, Grad-CAM, and Saliency Maps – to improve the interpretability and reliability of deep learning models used in plant disease detection. The aim is to analyze how combining multiple explanation methods can provide a richer and more comprehensive understanding of model predictions, aiding in the diagnosis and management of plant health. The developed approaches will be validated on state-of-the-art plant disease detection datasets, e.g., plant_leaves, PlantVillage, and plantae_k , as well as on a custom dataset we constructed. This dataset consists of images annotated with a disease severity score for wheat, enabling us to replicate human operator evaluations. The scoring system ranges from 1 to 9, capturing the severity of diseases such as wheat leaf rust and septoria tritici blotch
Compétences déployées
- Investigate whether finer representations of ensemble XAI are improving model insights.
- Assess whether a better understanding of decision-making processes can enhance model generalization—a critical challenge in real-world use cases.
- Explore whether improved decision comprehension can enable the integration of scores/images from different teams or datasets collected across different years. This is particularly important as human operator annotations may vary over time, with consistent criteria but fluctuating scoring practices.
Groupe de travail
Plaine
Labos / organismes
IRIT
Équipe d’accueil
Systèmes d’Informations Généralisés (SIG)
Collaboration / partenariats
INRAe / CIRAD
Encadrants
Moncef GAROUANI
Mathieu ROCHE
Jordan BERNIGAUD-SAMATAN
Tutelle gestionnaire
UT1
Dates stages
A partir de Janvier/février 2025
Formation
Informatique